Data Analytics: Focus On Your Why In The Age Of Big Data.
In this post, we’ll help you avoid an all-too-common pitfall in research: putting your “how” before your “why.” With the rise of “big data,” the number and kinds of analyses that are possible has grown exponentially. This growing focus on data analytics can tempt even the most capable researchers to focus more on how data will be analyzed rather than why it is being analyzed. In this post, we’ll provide a basic framework for identifying your “why” and making sure that you choose the right analysis for the job.
Identifying your “why”
If you find yourself in a situation where data and methods have been put before the purpose of the analysis, take a moment to ask “What problems are we trying to address?” and “What questions need to be answered to address them?” These two components (i.e. the key business problems and research questions) are what we consider the “why” of an analysis. Some examples:
Problem: “Our marketing efforts are not working as well as they could be.”
-> Key Questions You Might Ask: What are our most/least effective media channels? Is ad spending more or less effective in different regions of the country?
Problem: “We need to raise NPS.”
Key Questions You Might Ask: What affects a person’s likelihood to be a promoter? What are pain points in the customer experience that keep people from becoming promoters?
Problem: “We need to increase conversions through our digital channel.”
Key Questions You Might Ask: What aspects of our digital platform drive people away from conversion? What drives people to/from our digital platform?
Choosing the right “how”
Once you’ve defined your why, the next step is identifying the how – in other words, the data and methods you will use. With regard to data, a question like “What are our most/least effective media channels?” will require data that includes media spend by channel, as well as some measure(s) of effectiveness like sales, clicks, or donations.
When identifying your method of analysis, it’s important to remember that there are several ways to answer the same question and, often, simpler is better. Also, you’ll need to be sure that the data you have can support the analysis you will conduct. While the details of choosing and conducting an analysis are beyond the scope of this post, below are some examples of possible analyses that might be used to answer the questions above.
Question | Data You’ll Need | Possible Analyses |
---|---|---|
What are our most/least effective media channels? | Monthly spend in each channel as well as monthly outcomes in each channel (e.g. sales, clicks, etc.) | Basic: Compare outcomes in months with high, low, and no spend using descriptive statistics (i.e. means, percentages, correlations, etc.) Intermediate: Examine relationships between spend and outcomes while “controlling” for other factors like share-of-voice or time of year using some form of regression Advanced: Assess the total month-over-month impact of ad spend using time series analysis with lagged predictors. |
What affects a person’s likelihood to be a promoter? | Survey data that includes respondents’ likelihood to recommend as well as satisfaction ratings of their experiences with your brand/product/service. | Basic: Compare differences in satisfaction scores of promoters to non-promoters using descriptive statistics Intermediate: Look at relationships between each area of satisfaction and NPS using regression analysis Advanced: Use structural equation modeling/mediation analysis to identify possible intervening processes between satisfaction and NPS |
What aspects of our digital platform drive people away from conversion? | Web traffic information for each phase of the sales funnel (i.e. via Google Analytics) | Basic: Identify points in the sales funnel where potential customers drop off most frequently using descriptive statistics Intermediate: Explore the relationship between things like engagement time on certain pages and conversion using regression-based analysis Advanced: Use machine learning/predictive modeling to identify potential customers who are likely to convert but who do not and compare their engagement patterns to those who converted |
By following these steps, you’ll ensure that you avoid putting your “how” before your “why.” Getting this part of the analytics process right will reap rewards in both time and money spent, but perhaps most importantly, it will help you derive more robust, actionable insights.
If you’d like help getting the most out of your data, feel free to reach out to us at robertv@phase-5.com.
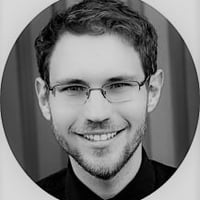
Author: Robert Vagi
Robert Vagi, PhD, is Phase 5’s lead data scientist. Drawing from his background in both quantitative research and education, Rob is passionate about helping clients use data to tackle their most challenging business problems. Throughout his career, he has helped clients in both private and public sectors make the most of their data. This work has received both local and national media attention. Rob is based in Minneapolis, MN.